Large Language Models with Multi Agents. (LLM-MA).
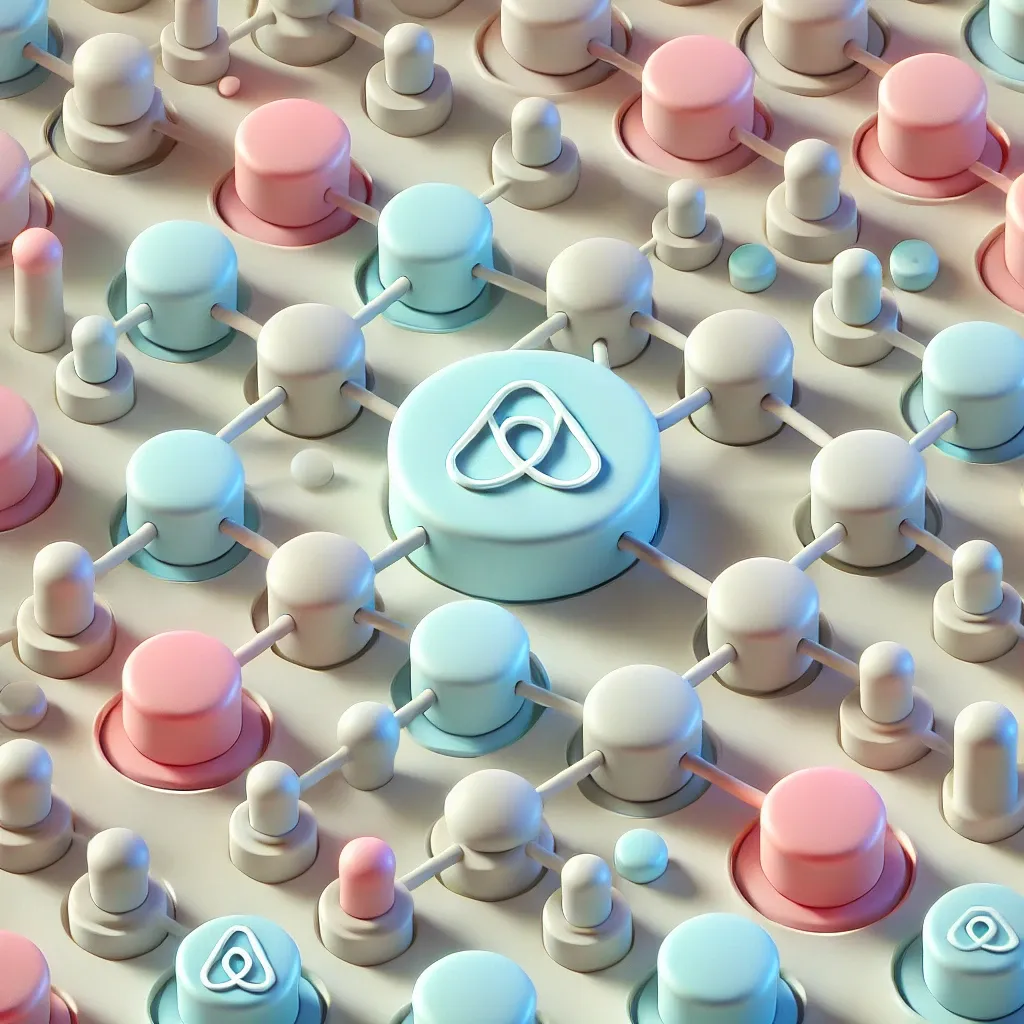
By: Mark Heather. Large Language Models (LLMs) have achieved remarkable success across a wide array of tasks. Due to the impressive planning and reasoning abilities of LLMs, they have been used as autonomous agents to do many tasks automatically. Recently, based on the development of using one LLM as a single planning or decision-making agent, LLM-based multi-agent systems have achieved considerable progress in complex problem-solving.
Large Language Models (LLMs) have recently shown remarkable potential in reaching a level of reasoning and planning capabilities comparable to humans. This ability exactly aligns with the expectations of humans for autonomous agents that can perceive the surroundings, make decisions, and take actions. Hence, LLM-based agents are able to understand and generate human-like instructions, facilitating sophisticated interactions and decision-making in a wide range of contexts.
LLM-based Multi-Agents are available to leverage the collective intelligence and specialized profiles and skills of multiple agents. Compared to systems using a single LLM-powered agent, multi-agent systems offer advanced capabilities by 1) specializing LLMs into various distinct agents, each with different capabilities, and 2) enabling interactions among these diverse agents to simulate complex real-world environments effectively. In this context, multiple autonomous agents collaboratively engage in planning, discussions, and decision-making, mirroring the cooperative nature of human group work in problem-solving tasks. This approach capitalizes on the communicative capabilities of LLMs, leveraging their ability to generate text for communication and respond to textual inputs. Furthermore, it exploits LLMs’ extensive knowledge across various domains and their latent potential to specialize in specific tasks.
Single-Agent systems empowered by LLMs have shown inspiring cognitive abilities. The construction of such systems concentrates on formulating their internal mechanisms and interactions with the external environment.
Conversely, LLM-MA systems emphasize diverse agent profiles, inter-agent interactions, and collective decision-making processes. From this perspective, more dynamic and complex tasks can be tackled by the collaboration of multiple autonomous agents, each of which is equipped with unique strategies and behaviors and engaged in communication with one another.
So, from a time and cost saving perspective, the recruitment process for onboarding an employee would mean sifting through around 20 CVs, interviewing 5 candidates, reducing them down to a final 2 and then making an offer. This would take at least 8 weeks to complete. You then train the new hire in your processes and procedures, probably another 4 weeks. It would then take them a further 6-8 weeks to become productive. A total time of around 6 -9 months. At an average salary cost for an employee in Cybersecurity being $100K that’s a $50K investment to get the new hire to start to pay for themselves. $50K + 6 months.
An AI agent is effective, in around 4 weeks including tuning the agent at a cost of $5K.
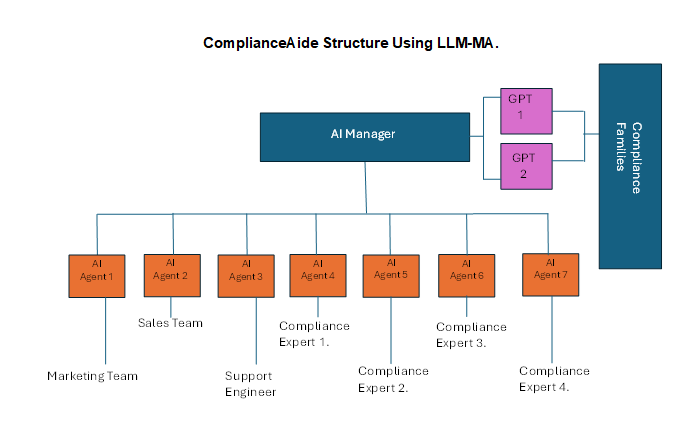
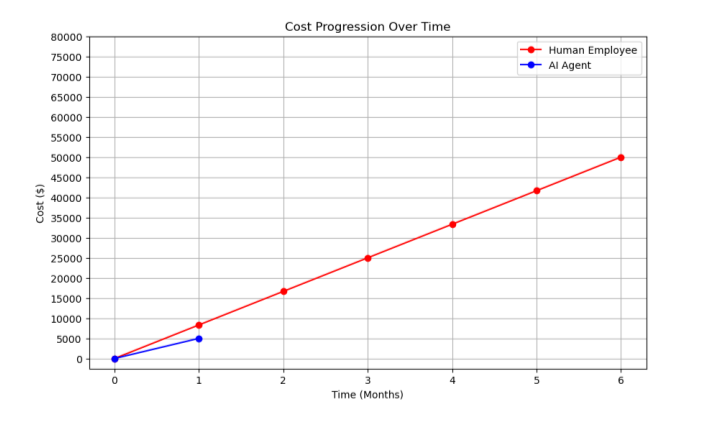
The red line represents the cost progression for a human employee, starting at $0 and reaching $50,000 at the 6-month mark. The blue line represents the cost progression for an AI agent, starting at $0 and reaching $5,000 at the 1-month mark.
If you need any further adjustments or have other questions, feel free to ask!
The red line represents the salary progression of a human employee, starting at $0 and reaching $50,000 at the 6-month mark. The blue line represents the salary progression of an AI agent, starting at $0 and reaching $5,000 at the 1-month mark.